Deciding on a Halloween costume can be tough, especially when your friends have taken all the good ideas already. How many more Harley Quinn costumes can a party have?
In 2018, researcher Janelle Shane released a machine learning experiment to solve this very issue. She trained a computer neural network to generate Halloween costume ideas.
AI costume generator
Artificial neural networks are a facet of artificial intelligence (AI). They are machine learning algorithms that extract meaning from large data collections, or datasets, to make decisions. Results vary depending on the complexity of the task. Neural networks (NNs) are used in a wide range of technology from rideshare apps to YouTube suggestions. So if NNs can get you an Uber, surely it can pick a Halloween costume, right?
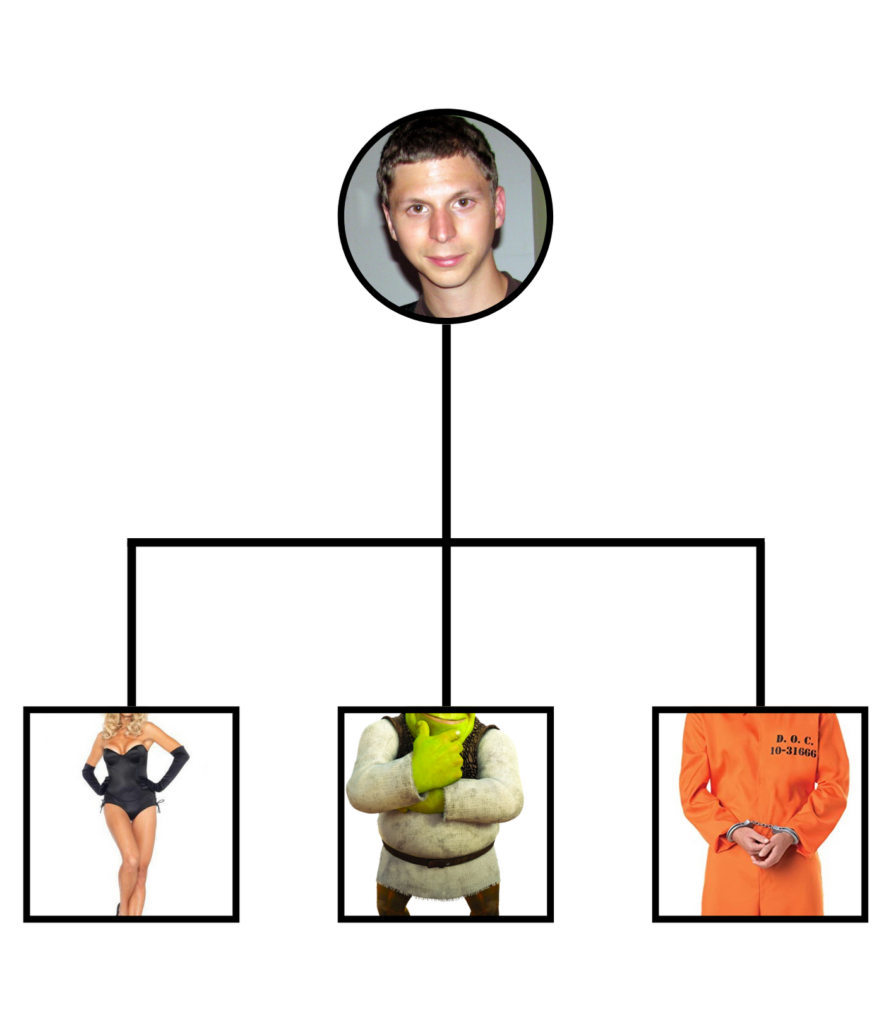
Shane puts this question to the test. Through 4,500 Reddit submissions, she created a dataset of numerous Halloween costume ideas like witches, vampires and hundreds of costumes with the word sexy in it. (Sexy poop emoji, sexy Cookie Monster and so much more.) Some suggestions were more bizarre, like Fungus Fairy Princess and Deadpool on a unicorn. The range of the dataset is random and creative, making the task of creating a costume quite tricky for an NN. To make the results as weird as possible, Shane decided to use an NN that learns words letter by letter with no knowledge of the word’s meaning.
The results were magnificent. Costume suggestions ranged from Shark Cow to Professional Panda to Vampire Big Bird. Other notable ones were Professional Panda and Sexy Michael Cera. These predictions were churned out of a two-step process: first, the NN predicts which letters should be used lexicographically to make the costume suggestion. Then it compares its prediction with the dataset it was fed. Every time there is a failed match, it refines the steps to make more accurate predictions.
The limitations of AI
Shane is the author of You Look Like A Thing And I Love You, where she writes about the funny, weird and spooky ways machine learning algorithms make incorrect decisions. Shane’s Halloween costume AI gives us an interesting insight into the limits of AI generally.
One constraint of AI-generated Halloween costumes is the lack of creativity. While Shark Cow might be the best idea you’ve heard in a while, the NN cannot work outside the scope of its training data. It can’t generate a suggestion from the latest news, trending topic or Netflix show. Also, the suggestions aren’t built off the meaning of words, but from the most compatible match within its dataset. This is what makes algorithms so limited and yet so eerie: It is very difficult to tell how they can come up with Lady Garbage without knowing what either word means. This grey area of unexplainable AI systems and decisions is called a black box, and for the most part, making predictions without trying to understand the reasoning is strangely accepted.
AI isn’t just used for funny Halloween costume generators. NNs are used in decision-making across all spectrums from convenience-based apps to loan eligibility to examinations and job hiring. The AI Ofqual scandal in the United Kingdom, where thousands of students were scored lower than their predicted grades for A-levels thus losing out on university offers, is just one harrowing consequence of the way algorithms work.
We should be wary of trusting decisions we can’t explain ourselves. NNs learn from the training data humans collect. What happens when those who collect the data, or the dataset itself, is biased? YouTube suggestions can sometimes be a maze of random videos and nothing like the videos in our history. If NNs can mess up something as simple as that, how can we trust a decision for loan eligibility, job qualifications or exam results? As Shane writes in her New York Times (NYT) article, “when we are using machine-learning algorithms, we get exactly what we ask for — for better or worse.”
Over the past few years, rapid development in AI has led to several ethical consequences. One example is with applicant tracking systems (ATS): an automated resume AI that reads thousands of resumes against a dataset of job requirements and internal business hiring patterns. What happens when every employee hired for one position is white or has an English name? Those who don’t match the data profile (i.e. white with English names) get immediately pushed aside and told someone better qualified has been selected.
“Neural networks are no more intelligent than humans — the algorithm will simply imitate the bias of its human controller.”
Shane also writes in her NYT article that, “we didn’t ask those algorithms what the best decisions would have been. We only asked them to predict which decisions the human in its training data would have made.” In other words, NNs will simply imitate the bias of its human controller.
The future of costumes
As digital transformation becomes reality, holiday costumes may be just another thing getting an automation makeover. Maybe the lesson of Shane’s Halloween AI isn’t that you should dress up as Vampire Big Bird for Halloween. Maybe the lesson is not to expect what we create with AI to be any better than what we create on our own.
We cannot hold artificial intelligence to a moral standard that we ourselves do not uphold. We also can’t expect it to be impartial when we aren’t. It is too soon to tell whether Sexy Michael Cera will become a cult classic, but going forward, we should be mindful of the elusive algorithms that are making decisions, and whether those decisions are the best possible outcome.
Recent Comments